The Challenge
In the railway network that the customer operates, the train frequency is high: at some stations, trains are leaving every 3 minutes. Therefore, a small disruption in the train network impacts the punctuality of many following trains. The task of the train dispatchers is to solve conflicts in the network efficiently, and with as few actions as possible, thus reducing the delay. The challenge is to build a tool that identifies conflicts in the network before they arise and recommends actions to solve those conflicts, supporting the tasks of the train dispatchers. In this innovative project, Eraneos’ data scientists, machine learning engineers and data engineers are supporting the customer’s development team to provide their expertise and contribute to the development of AI solutions.
Aimed at reducing train delays and improving network efficiency, one of the projects developed by Eraneos is a Digital Twin of the customer’s railway network using SUMO – Simulation of Urban Mobility, an open source package created by the German Aerospace Center, which is able to simulate urban transportation such as trains, trams, busses, and passengers in high detail on metropolitan scale.
The approach
In cooperation with the customer, the German Aerospace Centre (DLR), and experts from other consulting companies, Eraneos supported the creation of a Digital Twin simulation of the customer’s railway network using SUMO – Simulation of Urban Mobility. Among other things, Eraneos supported the customer in developing data processing pipelines and building Reinforcement Learning algorithms and applying them in real-time scenarios.
Reinforcement Learning algorithms such as Proximal Policy Optimization are employed within a multi-agent system. These agents observe scenarios within the network and respond by selecting actions to be executed within those scenarios.
In 2022 alone, around 58,000 minutes of delay could be avoided. That’s around 159 minutes a day; a significant improvement in the tightly scheduled railway networks.
In the event of a delay, the self-learning agents can recommend an action to reduce the delay in the network, for example, recommending that a delayed train wait for a punctual one before the two tracks on which the trains are travelling merge. The agents are trained to make the best recommendations to minimize disruptions. A baseline has then been developed that looks at how an actual dispatcher would react in each situation, so that the decisions of the reinforcement learning agent can be compared, and the dispatcher can improve his decision.
Every two minutes the reinforcement learning system runs a simulation of the whole network predicting the traffic flow one hour into the future, processing information from all the trains which are running, such as their location on the tracks, the estimated time of arrival and departure at the next stops with the goal of optimizing the traffic flow in the network. When a conflict arises that can lead to a delay, the RL agent determines what is the best action. The developed tool is used to communicate the recommended action to the train dispatcher. Then the dispatcher can evaluate the recommended action and decide whether the action should be executed.
The customer is a national railway company and one of the biggest railway operators and infrastructure owners in Europe. The customer wanted to further expand the use of Artificial Intelligence. In this project, AI is used to support the work of the train dispatchers and to increase punctuality of trains and improve the experience for travelers.
The result
In 2022 alone, around 58,000 minutes of delay could be avoided. That’s around 159 minutes a day and means a significant improvement in the tightly scheduled railway networks. This scales enormously, considering that the simulations influence millions of movements annually. This way, the network of the customer is gaining stability despite the restrictions imposed by construction work to modernize the routes. Due to the excellent results from the pilot project, the customer is now also using the technology on other networks as well.
The AI processes the current operating situation in a matter of seconds based on approx. 500 pieces of information per minute and generates its suggestions based on this. Based on live operation, it constantly simulates the development of the traffic situation and reports possible conflicts at an early stage. The dispatchers can thus intervene before a disruption occurs. As a result, traffic flows better: trains are less likely to have to slow down or wait when another train is blocking a section of the track. The improved flow of traffic can reduce delays by up to three minutes, and even by up to eight minutes during the morning rush hour. The customer can then make better use of the existing infrastructure and improve traveler experience significantly.
Let’s create sustainable change together
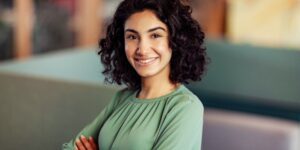
Contact our experts
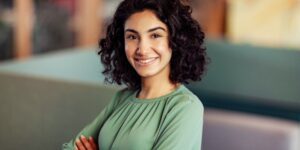