The Challenge
Efficient and prompt resolution of claims is not only vital but also expected by policyholders. It is one of the biggest challenges that claims professionals have in the insurance sector. Addressing this pain point leads to higher customer satisfaction levels and eases the burden on employees. For the past two years, our customer, one of the largest insurance firms in Europe, has been struggling under the weight of a growing claims backlog and a lack of data transparency.
The customer’s Operational Claims Management (OCM) team was under significant pressure as a result of its claims backlog while simultaneously trying to get to grips with an internal system migration. This meant that, for a period of time, the team was working across two different systems for handling claims. Altogether, the processing of claims was taking too long, resulting in customer dissatisfaction and heightened frustration for the claims team.
At the start of Eraneos’ engagement with the customer, there was an estimated backlog of around 100,000 claims and no transparency into what was driving it. Was it the type of claim, the system migration, or something else? Even when the client began to see some progress in reducing the backlog, a closer examination of the claims data showed that it was simply because fewer claims were being made. The client had little choice but to take a stab in the dark regarding its claims management.
“From start to finish, the entire project took around four months. We were able to uncover issues with standardization and greatly streamline claim resolution, saving time and increasing customer satisfaction.”
The approach
The approach we took for this customer was two-fold. The first step was to perform a root-cause analysis to identify pain points. The second was creating a forecasting model that assessed how long the processing of certain claims took, how many process steps were involved, and whether there was something particular in terms of their complexity regarding the cases that were sitting in the claims backlog.
Following our analysis, we developed an AI Model for Insurance Claims Complexity Assessment. The new AI model analyzed process logs to identify typical processing patterns and classify claims accordingly. The aim was to forecast workload and the specific needs of employees in various skill categories. This tool also identified cases suitable for fast-lane processing, enhancing claims management efficiency.
Our model analyzes large volumes of historical data to derive and evaluate typical processing patterns by complexity. It also identifies drivers of backlogs. Using known attributes at the time of claim creation, the AI model predicts expected processing patterns and identifies cases for fast-lane processing. For ongoing cases, the model incorporates ongoing progress into its predictions. The AI model is constantly evolving, with individual case forecasts aggregated into an overall workload forecast over time.
The customer is a large European insurance company, offering insurance, investment management, and financial services worldwide.
The result
The first major benefit of the project was an increase in transparency of how claims were managed. Our analysis found that the way claims were being processed was completely non-uniform and that better access to claims data would make it possible for the customer to predict the distribution of claims on a monthly basis. They could then make more accurate decisions on upcoming workloads and the required skill level of any OCM team member assigned to a particular claim.
Our AI model led to the successful prediction of processing patterns for claims using the information available at the point of case creation. By analyzing processes and clustering cases according to type and required workload, we were able to create a case management model that was three to four times more accurate than suitable dummy classifiers.
From start to finish, the entire project took around four months for Eraneos to complete. We uncovered the lack of standardization within the customer’s claims management process, found process quality issues, and greatly streamlined claim resolution to deliver higher levels of customer and employee satisfaction.
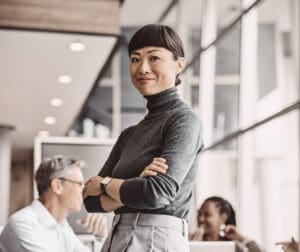