How can historical data and trend figures be leveraged to enhance the efficiency of marketing budget spending? A global FMCG company selected Eraneos to develop a solution that enables the incorporation of data, including both proprietary and Nielsen data, into the allocation of marketing budgets. This collaboration resulted in the creation of a scalable data hub solution that can rapidly process new data and assist various teams within the organization in making more informed decisions.
“Our client is a large international organization with numerous business units and departments,” stated Mallory Brickerd, Machine Learning Engineer at Eraneos. “Our primary point of contact was their Insights & Analytics department. They were particularly interested in understanding where marketing budgets could be deployed most effectively, particularly for new products. The client had data, including their own historical data and data from Nielsen, but it was not yet integrated or structured to provide the desired insights. “One of our initial objectives was to establish a robust platform for efficiently processing and analyzing the data.”
The foundation: a scalable data platform
The foundation of the project was the creation of a scalable data platform that would allow for the integration of both internal and external data sets, including data from Nielsen. This platform provided the foundation for analyzing the data and developing predictive models to support marketing decisions, such as more effectively allocating marketing budgets by product category and region. As an illustration, they were able to direct campaigns to products where they were confident demand would increase, significantly enhancing the impact of their marketing efforts.
“We handle thousands of products and a vast amount of data,” Mallory states. “Parallelization was therefore a crucial element in our approach. Without this step, the results would take an exceedingly long time to produce. By processing the data in parallel, we were able to significantly accelerate the prediction process and generate results in real time.
To process the substantial data volumes efficiently, a hybrid solution was developed, utilising Scala for data ingestion and PySpark for modelling. Furthermore, Azure Data Factory was leveraged to automate the process and guarantee that as soon as new data became available, it was immediately processed on the platform. This ensured that the solution remained scalable and flexible, even with the constant flow of new Nielsen data.
“The project demonstrates how a robust data infrastructure and strategic technology choices can facilitate data-driven decision-making.”
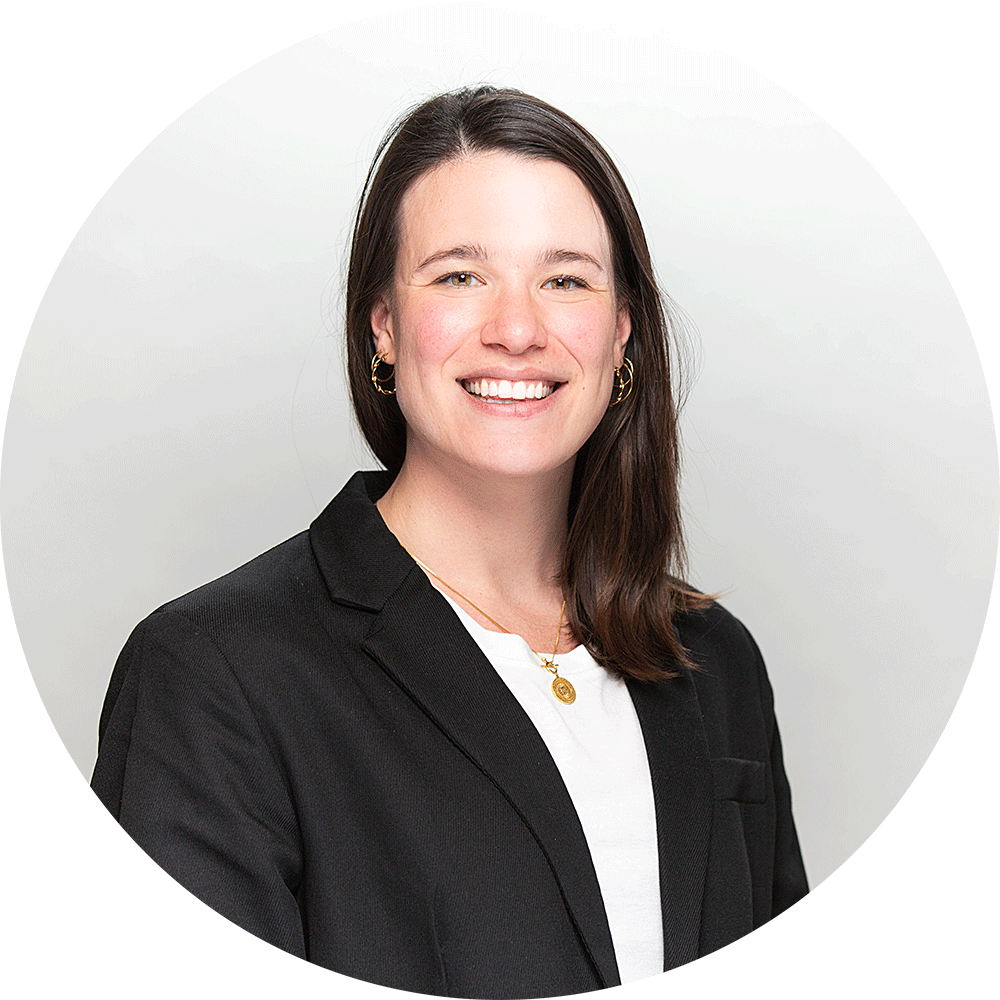
Stakeholder Collaboration: From Data to Actionable Insights
The success of a data-driven solution depends not only on the technology, but more importantly on its usability for various stakeholders. For the client, a large organization with diverse business units (such as health and hygiene), it was crucial that the insights from the predictive models had broad applicability. The diverse needs and priorities of each department, region, and team required a flexible approach.
“Developing predictive models is one thing. Making those models usable across teams and departments is another.” We began by providing product-level forecasts, but soon discovered that different stakeholders had disparate requirements. Some teams, like the one in Brazil, requested data organized by region instead of by product. This required the establishment of reports that could be readily aggregated in accordance with the specific requirements of each team. This flexibility was a crucial factor in the success of the solution.
Moreover, maintaining direct contact with the stakeholders was of paramount importance. At the outset, we primarily interacted with a single point of contact, who relayed the requests of other departments to us. However, we identified instances where details were misinterpreted during the translation process. Due to the positive outcomes, an increasing number of stakeholders expressed interest in utilizing our tools and forecasts. This prompted us to engage with other teams and stakeholders. They proposed new inquiries and sought to utilize the data in alternative ways. Our flexible approach enabled us to more effectively align the solution with their requirements, enhancing the efficacy and user-friendliness of the models and reports.
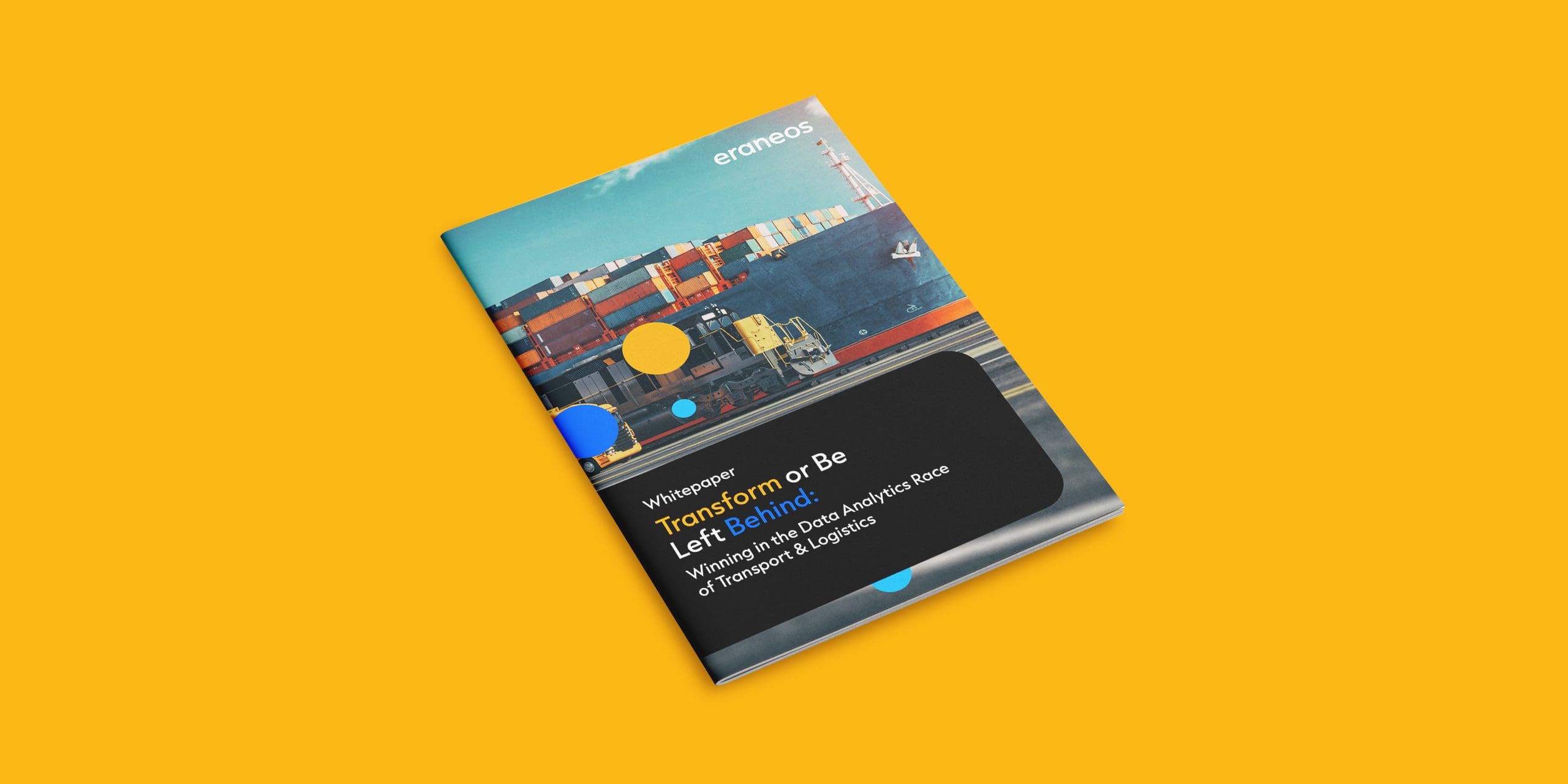
Winning the Data Analytics Race in Transport & Logistics
Learn how Data Analytics is empowering companies in the Transport & Logistics sector to remain relevant and gain competitive advantage.
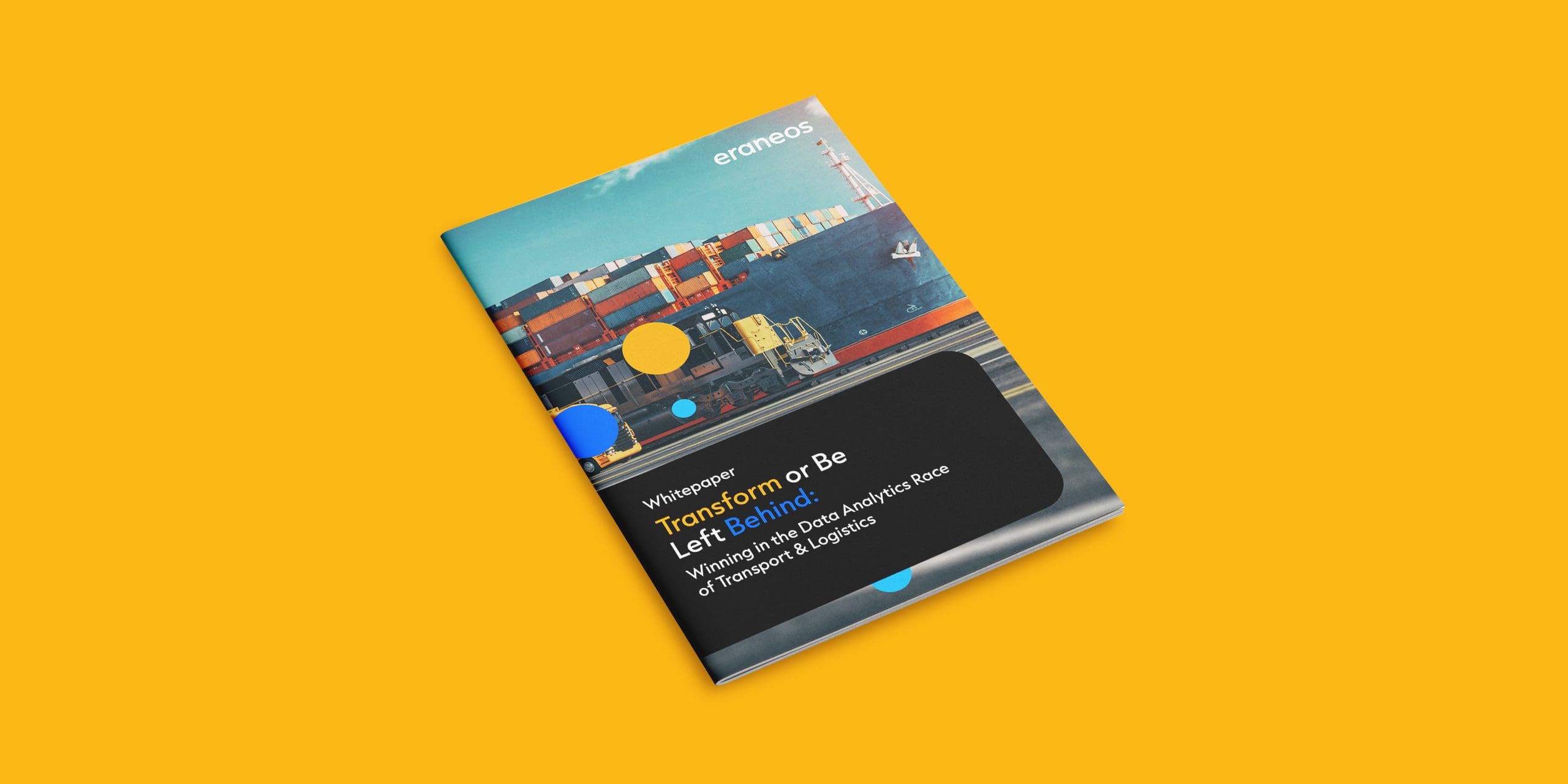
Applicable to other markets and customers
The project with the FMCG organization provided valuable insights for Eraneos that are now more widely applicable to other clients in other markets. The scalability and flexibility of the data platform developed provide a model for future collaborations.
“This project was my introduction to the capabilities of Azure. We identified the optimal technologies, including Scala, PySpark, and Azure Data Factory, to develop a robust and scalable environment. This approach is not only suitable for companies active in FMCG, but can also be easily applied to other customers with similar challenges.
The project demonstrates how a robust data infrastructure and strategic technology choices can facilitate data-driven decision-making. Through our collaboration with Eraneos, the client now has the tools to make accurate predictions about product sales and use these insights to further refine their strategy. Do you see potential opportunities, contact us to learn more about our capabilities and discover how we can help you create sustainable change.
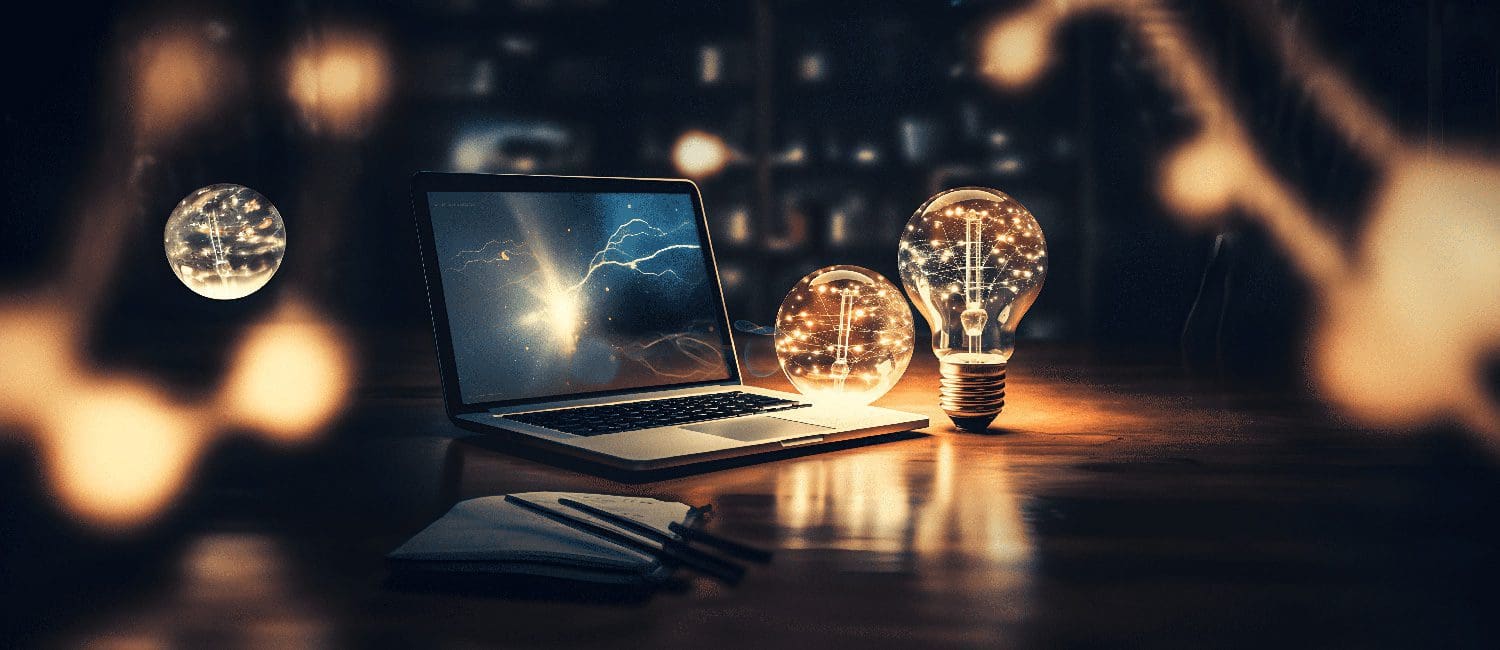
AI: Are you ready to go beyond Proof of Concept?
Want to know all about overcoming the challenges of implementing AI and achieving cross-functional success? Our AI and Innovation newsletter has you covered.
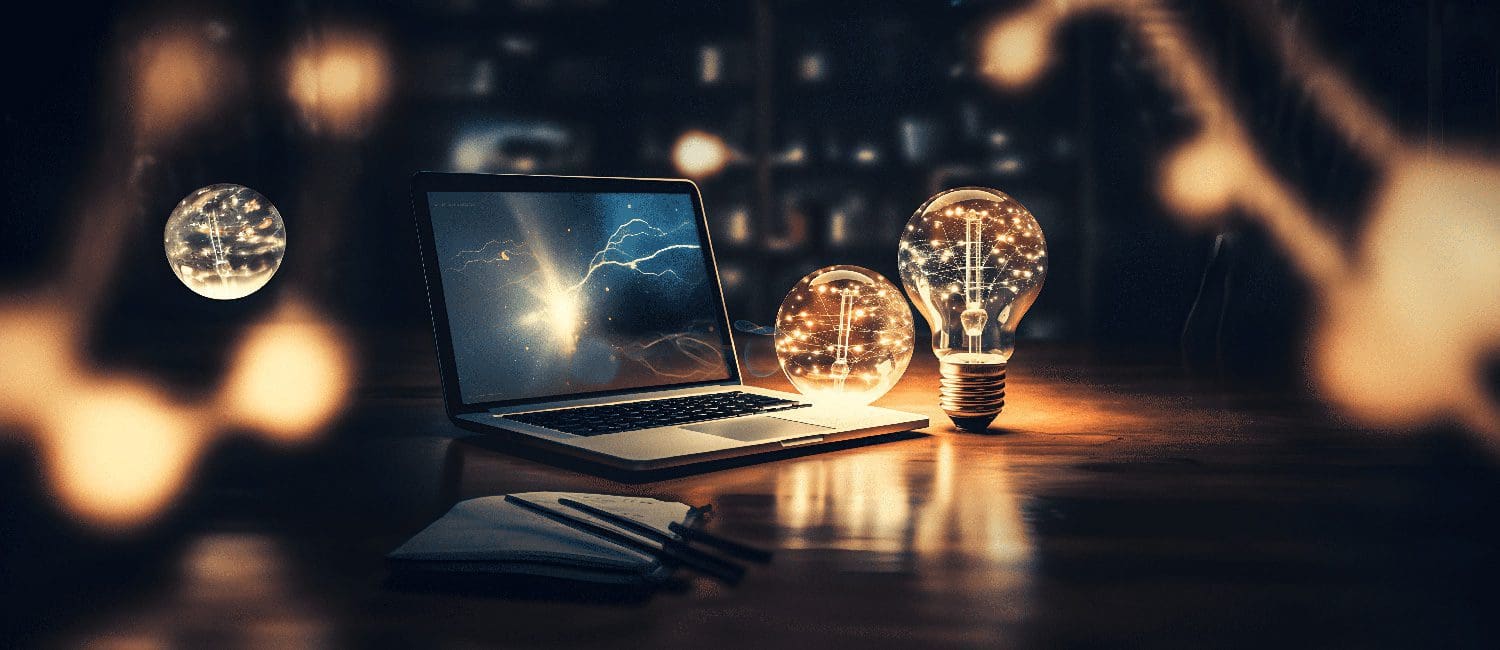